Credit underwriting has long been viewed as a behind-the-scenes process, relying on outdated systems and static data.
This was the case for decades, even as lenders experimented with automated algorithms and some self-serve options.
Fast forward to today:
- Open banking makes transaction data available in real time, revolutionizing access to financial behavior
- Transformers and generative AI make smarter, more contextual decisions—pinpointing profitable borrowers faster and more accurately than ever
- Real-time underwriting makes SMB lending both scalable and profitable. Previous lack of information and long processing times are now non-issues.
At Defacto, we’re putting this transformative technology to work: our underwriting model has scored over 40 million transactions, scored 250,000 companies, and originated 100,000 loans.
With real-time transaction data and a holistic view of borrower behavior, we’re increasing access to capital for SMBs while empowering banks and lenders to do the same.
Here's a deep dive into what we've built, how it evolved, and why it's going to change the SMB lending landscape.
The evolution of our underwriting model
2022: Traditional automated underwriting
Back in 2022, our underwriting model mirrored that of traditional lenders, relying on a handful of key performance indicators (KPIs) like a company's debt, growth, margin, and runway. This initial model was essentially an automated version of classic credit scoring—efficient but limited. The data was drawn from tax returns and public financial statements, much like what many lenders still rely on today.
While this approach let us onboard clients quickly and initiate loans, it was limited. Financial statements, prepared annually or quarterly, provide a snapshot that quickly becomes outdated. A company could experience significant changes in its financial health in between those statements, leaving us with a distorted picture.
Nevertheless, we made strides in automating the lending process. Our AI-powered model analyzes financial statements instantly, and we could initiate loans faster than any human-driven process.
But what came next is where things really got interesting.
2023: Transition to real-time transaction data
By 2023, we made a decisive leap from that traditional model to one based on real-time data and further enhanced by machine learning. Instead of relying on infrequent financial statements, our current model taps into real-time transaction and invoice data from a company’s financial tools. This lets us compute 130 specific KPIs to build a clear and highly accurate risk profile in real time.
For instance, we can analyze the average size of a company’s transactions and compare that to the amount of the loan requested. This level of analysis lets us assess a borrower’s capacity to repay in ways that static KPIs never could.
Our data sources now include accounting tools like Pennylane, direct bank connections through our Bridge API, and partnerships with services like Qonto, which allow us to access financial data with borrower permission.
The shift to real-time cash flow data is especially crucial for short-term loans, where timely information can make all the difference. The shorter-term your lending product is, the more important a client’s current cash position is.
If a company experiences a downturn, we want to know immediately—not months down the line when the next tax return is filed. Our ability to see the flow of revenue and expenditures allows us to understand not only what a company earns, but also its commitments, like payroll taxes and other debts.
And there are real positives for small business borrowers, too. Open banking data and AI in lending open new doors for SMBs, which are often rejected for loans due to a lack of available financial data.
By tracking these immediate financial signals, we’re empowering SMBs to access the funding they need without waiting for outdated reports to catch up.
The five key engines of underwriting
At Defacto, underwriting is a productized decision engine—not just an algorithm. We use five key engines—Data, Scoring, Decision Rule, Fraud, and Financial Product Factory—to deploy, backtest, and scale financial products with precision.
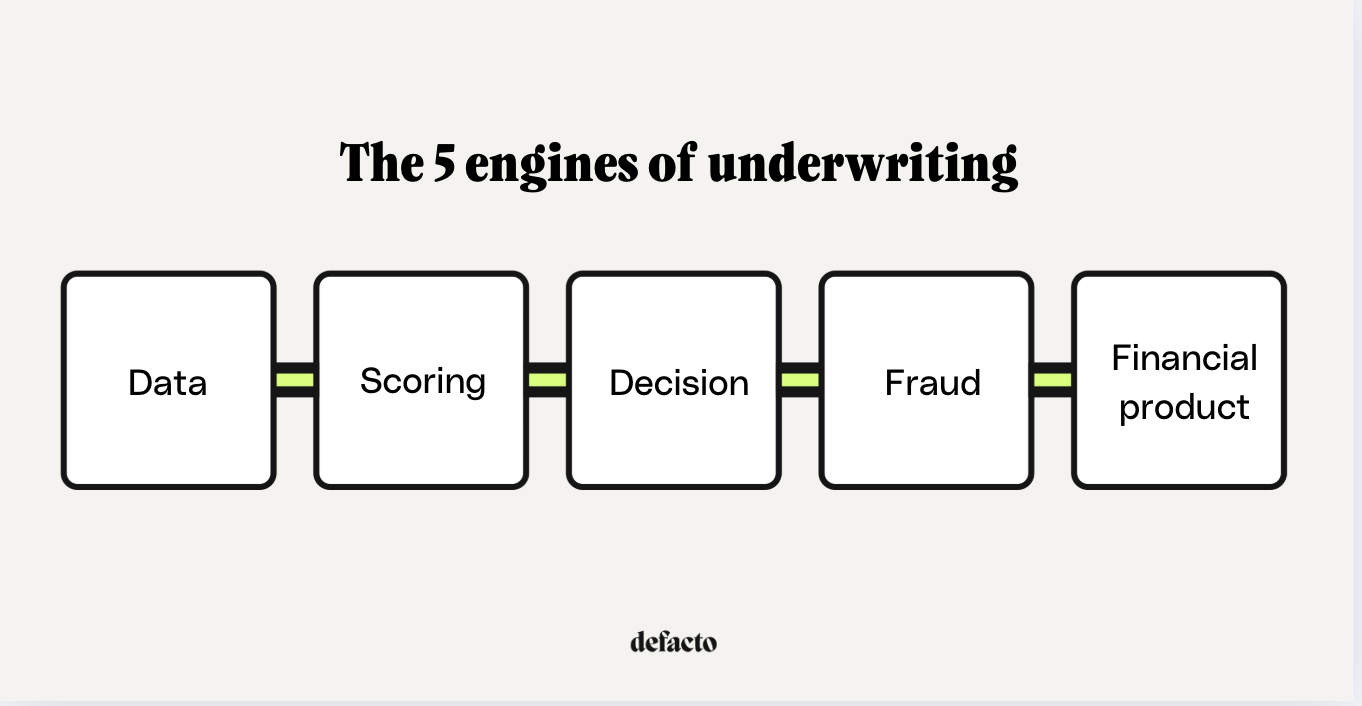
These five real-time services run in unison. They work independently, but each uses the insights and outputs from the others to inform their own decisions.
All five engines are crucial to transforming a traditionally manual, complex process into something fully automated. Here’s how they work.
1) Data engine
At the heart of our system is the Data engine, which aggregates public financial information and real-time transaction and invoice data. Here, we employ transformers and large language models (LLMs) to clean, structure, enrich and categorize this data, providing deep insights into borrower behavior.
2) Scoring engine
Our Scoring engine takes the rich, real-time data from the Data engine and uses it to build a comprehensive risk profile for each business. This engine employs sophisticated algorithms to analyze up to 40 individual metrics—everything from payment behaviors to transaction volumes—to assess the level of financial risk associated with a loan application.
By drawing correlations between various data points, the scoring system can provide a more holistic and granular view of risk, beyond what traditional credit scores offer.
And with real-time, transaction-level data, we ensure that every loan decision is informed by the most accurate, up-to-date financial picture.
3) Decision Rule engine (Defacto's underwriting tool)
The Decision Rule engine enforces a series of checks and audits to ensure that our decisions are sound. With 52 independent validation points, our framework assesses a company’s financial profile, from compliance to capital structure. These validation checkpoints are entirely independent, allowing for highly precise decisioning and transparency about why a loan may be stalled or declined.
If a loan application is flagged, our framework provides clear paths to resolution, ensuring that no potential loan is unfairly denied. Both the borrower and our own team can see precisely which requirements are not met, and can quickly follow up.
This approach adapts to individual applications—if one aspect of the model needs adjustment (such as incorporating new payment behaviors), we can do so without disrupting the entire process.
The system evolves with changing market conditions and regulatory requirements, while also remaining specific to each application. We cross-reference public data and compliance checks—including company ownership, capital levels, and late payment records reported to regulatory bodies like the Bank of France—to ensure comprehensive due diligence.
4) Fraud engine
Our Fraud engine is a centralized hub that detects suspicious activities across our platform, safeguarding both us and our clients from financial missteps. Thanks again to real-time transaction data, we can automatically detect suspicious transfers or unusual activity.
These could include:
- A user borrowing a sum and then quickly transferring that money to a private account, or spending it on something that’s not business related.
- Falsified or embellished invoices.
- Unusual suppliers or third parties to invoices, which wouldn’t normally make sense
- Unexpected costs that aren’t part of the company’s working capital requirements. For instance, a food producer seeking financing for a mobile phone invoice.
Our automatic detection and data collection let us spot these instances immediately and notify both the customer and our team. And even where we don’t have access to all of a company’s financial accounts, the system can automatically detect any gaps in the financial picture.
Invoice contextual analysis plays a vital role here. When a business submits an invoice for financing, the system checks whether the invoice aligns with the company’s operations and typical costs.
5) Financial Product Factory
Finally, our Financial Product Factory designs and deploys tailored financial products that adapt to the real-time data we collect. This ensures that our loan offerings meet the specific and evolving needs of each client.
Tangibly, this engine lets us make changes based on the real-time data we gather throughout the loan’s lifecycle. As a borrower’s financial needs for working capital, expansion funds, or cash flow optimization develop, each loan offering is perfectly aligned.
It’s a more dynamic, efficient way to do SMB loan decisioning. We rely on diverse data sources and continuous monitoring, rather than one-off meetings or quarterly check-ins.
This robust and agile framework ensures a smarter, more adaptable lending experience for both borrowers and lenders using Defacto Core.
Leveraging LLMs for enhanced decision-making
Integrating LLMs into our Data engine has been transformative. And the speed and accuracy of the whole underwriting model (and the five engines) is really only possible with AI and LLMs.
The amount of data available—and the speed in which it’s available—changes the lending process completely. But it’s only valuable if we can take these (almost) endless data points and derive meaning from them.
Manual, human processes could never do this at scale. And even earlier lending algorithms were far too limited, working only in sequence and with predictable inputs.
Thanks to transformer neural networks, underwriting models can draw from more disparate, seemingly unconnected data sources. We’re no longer limited to “if this, then that” logic.
LLM models validate and enrich the data we receive, while also informing our Decision Rule engine. They take financial information from customers, plus our own past decisions and results, and make credit decisions that benefit Defacto, our partners, and our customers.
The human checkpoints in credit decisioning
The majority of Defacto loan decisions—85% to be precise—are fully automated. Many borrowers check eligibility, supply data, agree to a loan, and onboard themselves with no human help. This has let us deliver over €600 million in loans with only one customer support staff member.
But in those instances where human input is required, the underwriting model makes this far more efficient.
We rarely decline a loan outright. Instead, the model flags all the potential risks or areas where we need more information and notifies a team member. This makes it very easy for that human agent to jump straight to the key issues and make a decision.
We want to serve edge cases, and the ability to update information as circumstances change. The model finds these instances for us, and it’s very quick for a team member to make the right call.
An ongoing learning process
Short-term lending requires dynamic decision making and constant improvement. Fast offers and quick resolutions mean lenders can iterate immediately and learn from each financing agreement.
This is a major positive of keeping human agents involved. We could remove the human element and let the model decline more applications outright. But we’d have no way to know how many of those declined loans would actually have worked out well in the end.
And it’s important to review the model and look for biased assumptions we’ve built in. There may be things that look like red flags at first, but actually never lead to risky loans or defaults.
Here’s a specific example: Negative cash in an account is an obvious concern. But in some cases, a company with -€50,000 in their account can be more creditworthy than one with only €1,000. That second company may have very limited financial flow.
Today, if a client’s account goes below zero, we can ask them for their overdraft contract. A large overdraft granted from a major bank is a real sign of trust in that business.
There are always new data points to add or lessons to learn along the way that help to improve our underwriting assumptions.
The advantages of real-time credit decisioning
When we say “real time,” we’re actually talking about two things:
- Using real-time data rather than outdated financial statements; and
- Providing a, instant response to the borrower—in real time
Both are hugely important. We’ve already seen the benefits of real-time transaction data for better decisioning.
But there are clear advantages to providing near-instant decisions to customers
- They can check their eligibility and explore financing options without sitting through a sales pitch or booking a meeting
- They can accept our proposal and receive funds right away, rather than shopping around
- They can resolve any issues in their application in real time
As a result, our clients benefit from faster onboarding, with access to funds in six minutes versus the industry average of 3 weeks. And our Core customers maintain better recovery rates (55% versus 40% industry average).
Credit underwriting to better serve SMBs
Lending innovation is exciting in its own right. But at Defacto we’re particularly excited about the ways in which automation and AI are transforming SMB lending.
These modern tools and approaches broaden the pool of eligible applicants, remove delays from traditional financial statements, and significantly boost underwriting efficiency. By making faster, more informed decisions, we reduce risk and deliver customized financial products that meet the unique needs of each borrower.
Our real-time, data-driven model solves the key funding challenges facing SMBs and positions us to lead the industry in reshaping how businesses access financing.